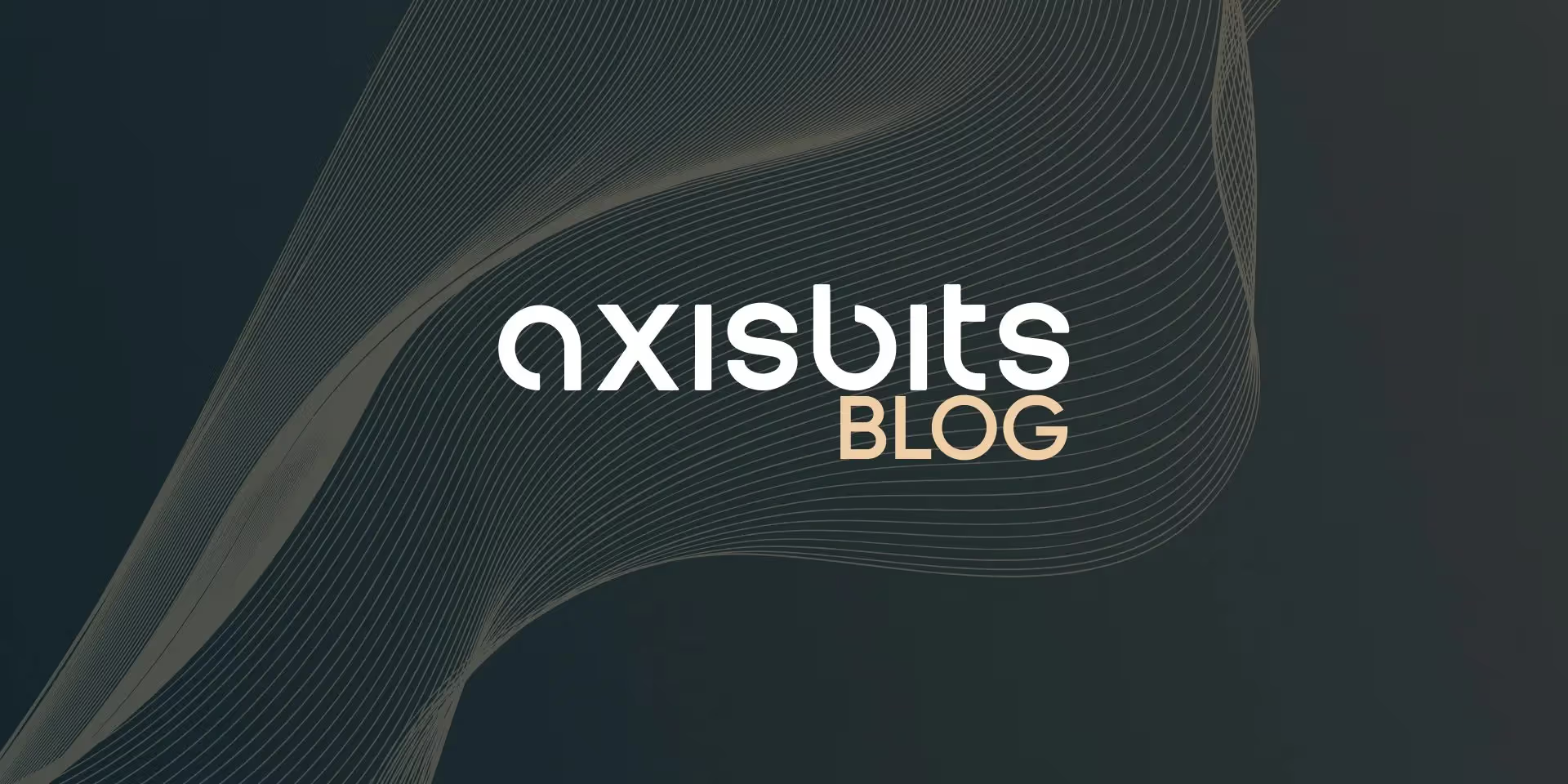
A capability for the prompt analysis of large amounts of digital data is a tool that largely determines the success of modern financial institutions. Any market participant needs to understand why the world's largest banks are investing heavily in this area.
To state the immediate answer - it is to increase its productivity and responsiveness, and also the efficiency and validity of the decision-making process. However, the benefits of adopting data science-driven automation do not stop there. In this article, we examined the top 9 data science use cases in the banking and financial industry.
Data Science in Finance
Big Data, AI, Machine Learning - these terms are linked inextricably with several data science disciplines aimed at ensuring the timely making of optimal business decisions based on processing previously unimaginable amounts of information in real-time.
The world's largest banks operate terabytes and even petabytes of valuable customer information every day. Refusing to use these data and the opportunities provided by their analysis means abandoning one of the critical development vectors. Banks' motive for employing data science in finance is clear: they strive to increase efficiency to the possible limits. The introduction of technologies based on data science provides:
- Increase in the loyalty of customers through personalized financial management and promotion of the offers most relevant for each of them;
- A well-developed system of data science risk analysis and prevention of fraudulent activities, capable of self-training;
- Higher profitability and productivity of operational processes;
- Increase in the efficiency of employee knowledge and skills development;
- Possibilities for tracking the productivity of personnel and monitoring "toxicity" within the team.
According to Celent, in 2019, banks invested $270 billion into developing their technology stacks and introducing innovations. It is expected that by 2022, this volume would grow to over $300 billion. Impressive numbers, are they not?
Top 9 Data Science Use Cases in Banking
Let us analyze the most important cases for the introduction of data science in banking and finance. Keep in mind that many of these cases are interconnected and co-dependent.
1. Risk analysis management
Risk management is one of the most critical aspects of the provision of financial services. This discipline is vital to the safety, reliability, and profitability of a company's day-to-day operations.
A variety of risks arises from the need to interact with competitors, investors, regulators, customers, and other institutions. They differ in importance and the potential for monetary losses. Accordingly, the first steps in reducing risks within banks are identifying, prioritizing, and monitoring them.
By analyzing gigantic volumes of information on customers, loans, insurance results, and other market operations, modern algorithms based on machine learning and risk management data science methodologies independently improve risk assessment models, gradually increasing institutions' responsiveness and profitability.
2. Customer data management
By accumulating customer information, you can build a behavioral profile to determine the most appropriate sales promotion methods further. Having comprehensive information about the client and the history of the company's interaction with them, experts can identify significant trends and predict future behavior with a high degree of accuracy. Moreover, data science helps to automate this process and free up valuable employees' time to solve more critical and creative tasks.
3. Fraud detection
Machine learning algorithms allow timely detection and suppression of fraudulent operations related to bank cards, accounts, transactions, and so on. For example, you can highlight new accounts from which suspiciously expensive purchases are made. Banks are also implementing systems for monitoring abnormal transactions based on behavioral profiles. For example, if a customer suddenly orders a transaction that does not fit into their typical behavior, banking algorithms may request additional confirmation to complete it.
4. Personalization
Data Science for banking creates opportunities for organizing effective customer interaction through personalized marketing. By analyzing the array of accumulated information, self-learning algorithms compose individualized offers that will be most interesting for this particular consumer. In turn, the company benefits from expanding the range of offered products and services, as well as increasing sales.
5. Customer base segmentation
Segmentation helps you to serve multiple customer groups better. Groups are distinguished based on behavior and other principles, using logistic regression, clustering, etc.
6. Designation of customer lifetime value
The ability to assess customer lifetime value (CLV) at the very beginning of the interaction with them allows companies to move from focusing on quarterly profits to the customer relationship management strategy that has already demonstrated increased long-term profitability. Dividing customers into groups based on their CLV allows you to focus efforts on improving the quality of service and increasing overall staff productivity properly.
7. Real-time analytics
High quality real-time predictive data analysis allows businesses that use its power to track all changes in the market. Today, banks that do not skimp on the introduction of innovations track transactions, changes in credit ratings, new legislative initiatives, and thousands of other factors affecting market conditions and react almost instantly.
8. Monitoring customer feedback
Machine learning algorithms and techniques enable you to analyze customer experiences, creating a foundation to improve the effectiveness of service strategies and product offerings.
9. Automation of communication with clients and the expansion of communication channels
Mobile communications, social networks, e-mail, instant messengers - a successful company must communicate with its customers through any channels convenient for them. This is also true for financial institutions.
Chatbots, electronic assistants, expert systems – today, a wide variety of automated means of interaction with the consumer allows you to relieve the company's staff from routine tasks and increase communication efficiency.
Data Science for Banking: There are no Universal Recipes
Like any other company, when introducing innovations, financial institutions solve a set of internal tasks: collecting data, attracting specialists, building a technology stack, identifying effective marketing solutions - preparing all those plans and methodologies that will be implemented in the targeted business processes.
At the same time, it is difficult to say what will "play" in the case of a particular bank, and what will not justify the costs. How not to fail? t is necessary to design the processes initially in such a fashion so that it is possible to measure results clearly. It is crucial to define cost savings or profit increases relative to what was before the integration of new methods. Anyway, an economic model can be calculated in almost any case.
On average, data science tools show results in a short time frame (if you do not take into account direct infrastructure expenses). Analyze tools that can improve processes, try them, test hypotheses to see if they work or not. After that, decide upon the viability of launching the project.
Software Development VS Data Science
Software developers mainly create products in the field of data science, while data scientists analyze the data. Data science is nothing new, actually. For decades, humans have processed information using computers to predict changes in stock market trends, weather, and other phenomena.
The differences between software development data science and data science itself lie in the process, outcome forecasting methods, tools, and infrastructure. Does a data scientist need to be able to code? Not really.
Differences between Software Engineering and Data Science Projects
Software Engineering Data Science Project Feasibility Generally known upfront whether a project is executable Might not be known until late project phases Focus Delivering functioning software systems Delivering actionable insights Longest Phase Development (coding) Data preparation Scope Largely defined by stakeholders and product managers Somewhat define-able by stakeholders and product managers but also needs to be uncovered based on what the data scientists discover Task Estimation Task completion time is generally estimate-able The time required to deliver many steps are unknown Progress Tracking Somewhat definitive through metrics like number of features or story points complete More ambiguous. Example: Being 50% done with a model doesn’t mean anything Knowing it works Mostly binary. Software either works per the specifications or it does not (e.g. the user interface loads or it doesn’t) Many shades of gray. Given a model, one person can say it is working and another could say it is not. Both can be right given their frame of reference according to Data Science Project Management
Conclusion
In this article, we briefly reviewed data science use cases, which provide the fastest and most noticeable result, including the introduction into the banking sector. One thing is sure: the methodologies and tools offered by data science can improve the accuracy of risk management, customer service quality, as well as automate and accelerate many business processes, increasing the overall efficiency of the company. To keep pace with the times and increase profitability, it is important to adopt new methods and algorithms for working with information on time.